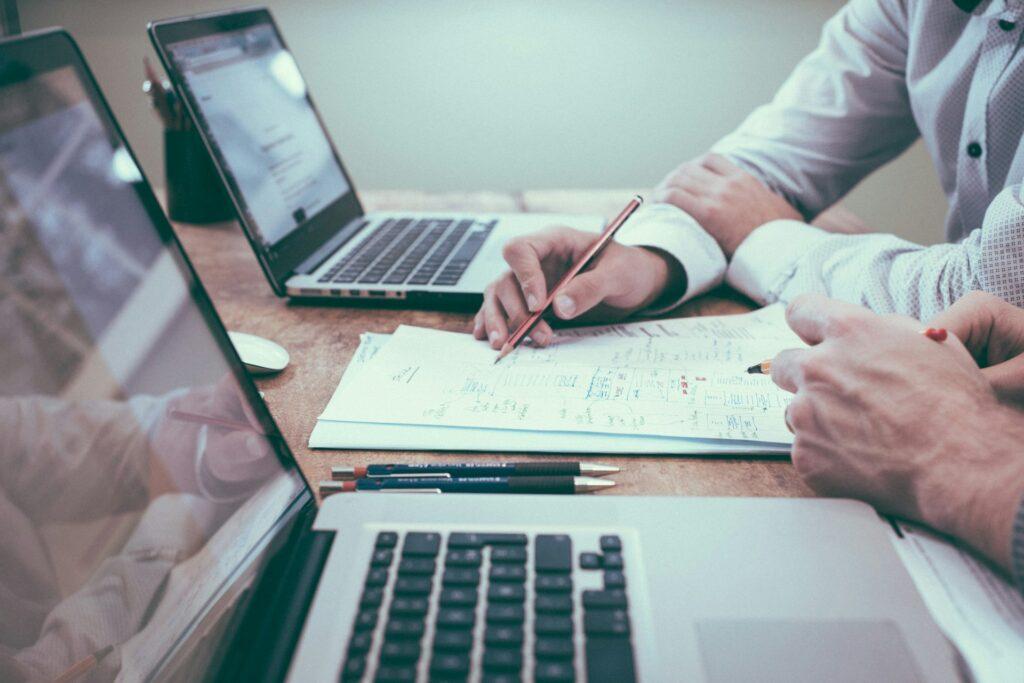
AI Data Analytics vs. Traditional Data Analytics
In the rapidly evolving landscape of data analysis, businesses are increasingly looking for the best tools to turn raw data into actionable insights. Traditionally, data analytics has been a manual, time-consuming process, but the advent of artificial intelligence (AI) has brought transformative changes. This article compares AI data analytics with traditional data analytics, highlighting the advantages of AI tools like Qmantic and their impact on modern business practices.
Table of Contents
1. Data Processing Speed and Efficiency
Traditional Data Analytics
Traditional data analytics relies heavily on manual processes and basic software tools. Data analysts must collect, clean, and process data before they can perform any meaningful analysis. This process is often time-consuming and labor-intensive, especially when dealing with large datasets. Moreover, traditional tools may struggle with handling big data efficiently, leading to delays and potential errors.
AI Data Analytics
AI data analytics, on the other hand, leverages machine learning algorithms and advanced computational power to process data at unprecedented speeds. AI tools can automate data collection, cleaning, and analysis, drastically reducing the time required to gain insights. For example, Qmantic uses sophisticated algorithms to quickly process and analyze vast amounts of data, providing businesses with timely insights that can be critical for decision-making.
Example: Qmantic’s Efficiency
Qmantic’s AI-driven approach can analyze complex datasets in minutes, allowing businesses to respond to market trends and operational issues faster than ever before. This efficiency not only saves time but also enables more agile and informed decision-making processes.
2. Accuracy and Precision
Traditional Data Analytics
Accuracy in traditional data analytics largely depends on the skill and experience of the data analysts. Human error, biases, and subjective judgment can affect the accuracy of the analysis. Additionally, traditional methods often involve simplifying assumptions that may not capture the full complexity of the data, leading to less precise results.
AI Data Analytics
AI data analytics tools are designed to minimize human error and biases by relying on data-driven algorithms. These tools can identify patterns and correlations that might be missed by human analysts. Furthermore, AI models improve over time as they are exposed to more data, leading to continuously enhanced accuracy and precision.
3. Predictive Capabilities
Traditional Data Analytics
Predictive analytics in traditional settings often relies on basic statistical methods and historical data. While these methods can provide useful forecasts, they are typically limited in their ability to predict complex, non-linear patterns. This limitation can hinder a business’s ability to anticipate future trends and make proactive decisions.
AI Data Analytics
AI data analytics excels in predictive capabilities by using advanced algorithms to model complex relationships within data. Machine learning models can learn from historical data and make highly accurate predictions about future events. These predictive insights are invaluable for strategic planning, risk management, and identifying new opportunities.
4. Scalability and Flexibility
Traditional Data Analytics
Scalability is a significant challenge for traditional data analytics. As data volumes grow, the manual processes and basic tools used in traditional analytics become increasingly inadequate. Scaling up often requires substantial investment in additional resources and infrastructure, making it a costly and time-consuming endeavor.
AI Data Analytics
AI data analytics tools are inherently scalable. They can handle large datasets efficiently and grow with the business’s needs. AI models can adapt to new data inputs and evolving requirements without the need for extensive manual intervention. This scalability makes AI analytics tools like Qmantic particularly valuable for businesses looking to expand and adapt quickly.
5. Integration and Usability
Traditional Data Analytics
Integrating traditional data analytics tools with existing business systems can be challenging. These tools often require extensive customization and manual input, which can hinder their usability and slow down the analysis process. Furthermore, the learning curve for traditional tools can be steep, requiring specialized knowledge and training.
AI Data Analytics
AI data analytics tools are typically designed with user-friendliness and integration in mind. They offer intuitive interfaces and automated processes that simplify data analysis. AI tools can easily integrate with various business systems, providing a seamless experience for users. This ease of use enables more employees to leverage data insights in their decision-making processes.
Conclusion
The comparison between AI data analytics and traditional data analytics highlights the transformative potential of AI tools like Qmantic. AI data analytics offers significant advantages in terms of speed, accuracy, predictive capabilities, scalability, and usability. By leveraging these benefits, businesses can make more informed and timely decisions, gaining a competitive edge in their respective markets.
Qmantic, with its advanced AI-driven approach, exemplifies the power of modern data analytics. Its ability to process vast amounts of data quickly, provide accurate and predictive insights, scale with business needs, and integrate seamlessly with existing systems makes it an invaluable tool for any organization looking to enhance its decision-making processes. As businesses continue to navigate the complexities of a data-driven world, the adoption of AI data analytics tools like Qmantic will be crucial in turning data into actionable insights and driving sustainable growth.